Farmers have battled weeds since the earliest days of agriculture and have traditionally used some form of tilling as weed control. That changed in the 1940s when synthetic herbicides became commercially available, and soon after, the first herbicide-resistant weeds took root. While stronger chemical formulas and widespread coverage were once effective weapons, over time, the weeds have been winning this war. Today, scientists are searching for new solutions in both modern technology and traditional practices.
Drs. Wes Lowe and Daniel Chesser, assistant professors in the Department of Agricultural and Biological Engineering, and Dr. Brian Pieralisi, assistant professor in the Department of Plant and Soil Sciences, all MAFES scientists, conducted a study funded by Cotton Incorporated to determine the capabilities of artificial intelligence and automated mechanical technologies in weed detection and control.
"In 2020, we were approached by Cotton Incorporated to design a tool to control herbicide resistant weeds in cotton production systems," said Lowe.
Weeds can become herbicide resistant as a result of using the same herbicide, or herbicide type, for several years. For any given species of weed in a field, a small number have a slight genetic difference that makes them resistant to the applied herbicide. Over time, the "survival of the fittest" process takes place, and those stronger weeds eventually become the dominant type in the field.
"Years of repeatedly applying the same mode of action herbicides and generations of exposure have led us to this point," said Pieralisi. "There are only so many chemical solutions available, and the problem just gets worse with more exposure to them."
Today, farmers have access to technology-driven weed control systems that eliminate the need for traditional broadcast spraying methods. For instance, John Deere's See & Spray uses a multi-camera system to identify and spot spray individual weeds, leaving crop plants and everything else in the field alone. While this artificial intelligence technology, or AI, has vastly improved weed control methods, it does not completely solve the problem of herbicide-resistant species.
"We wanted to draw from the See & Spray's AI model, but we thought that we needed a design that would identify and either till or spray, depending on the species," said Lowe.
Pieralisi added, "Our main goal was to control weeds while minimizing the impacts on the field environment. We wanted to till herbicide-resistant weeds and large weeds, which are hard to control with herbicides, and spot spray those without a resistance. We didn't want to till everything because we wanted to conserve as much soil moisture as possible and to conserve as much residual herbicide protection as possible by minimally disrupting the soil surface."
The tools available to farmers today are exclusively spraying or tilling systems. No one has developed a product that will do both simultaneously. The work these scientists are doing is truly groundbreaking, tackling the tough weeds while protecting the soil environment as much as possible.
The team began the project last year by identifying every plant growing in the spaces between rows of cotton crops, referred to in the industry as "green on brown." Then they tested their tillage system, a John Deere 845 cultivator outfitted with Trimble WeedSeeker optical sensors to trigger hydraulically-actuated tillage tines that plow the detected weed out of the soil.
During these trials, they discovered some limitations with the WeedSeeker system: it did not respond as quickly as necessary for their purposes, and it had difficulty with shadows and varying light conditions. The team needed a more precise optical system to identify the weed by species and determine whether to till or spray it. This year, they began working with an intelligent industrial camera made by IDS, a German technology company, and have seen improved results.
"As we give the camera more images of weeds, it learns to identify them and becomes more 'intelligent'," said Lowe. "We're currently working on building a robust collection of images in the lab and hope to take the camera out into the field soon."
The scientists have been training the camera in the lab by potting various weeds and running them on a conveyor belt underneath the camera, while graduate student Grace Friday classifies the images and helps train the system to detect more accurately. The lab setup simulates the AI camera moving across a field and teaches it to capture and identify the images at speed so it can quickly communicate with an on-board control system to trigger the appropriate method of control for the weed: spraying or tilling.
The team's initial plans for designing the tiller came from an Australian-based weed chipping system. However, pandemic-related supply chain disruptions prevented them from obtaining components from this tool. In response, the scientists developed a concept using old-fashioned row crop cultivators that farmers had left sitting for years - perhaps appropriately - in the weeds behind their shops.
"We thought that, if we could figure out how to retrofit the equipment, this would be an easily implementable and inexpensive solution for farmers," said Lowe. "We're not trying to reinvent the wheel. We want to see what else we can do with the wheel."
Farmers must make large financial investments in their equipment and hope that profits will make up for those upfront costs. A system that could retrofit existing machinery would allow them to benefit from the latest technology without having to take a great financial risk.
"Adapting existing equipment with new technology is a trend we're seeing in the AI and machine learning field," said Chesser. "Farmers are looking to this technology to alleviate labor costs and optimize their production systems. In the future, our tool could potentially be integrated into an autonomous system that would traverse a field by itself, further reducing labor costs."
Lowe stressed that more work is needed to refine this system, so a commercial product may be up to five years away. While current funding expires at the end of the year, the team has applied for financial support to continue the study into the next year.
"We don't want to just throw the technology out there without further testing and refinement," he said. "But with the right support from industry partners, I think we could move this into something that could be used in a production system easily, filling a much-needed deficiency in weed control."
In addition to refining the mechanical and technological components of their device, the team hopes to develop a weed database containing images of multiple species taken from multiple points of view. A larger collection of images would further enhance the camera's capabilities of identifying species.
"A weed database organized by region would allow the camera to learn to recognize weeds prevalent in a specific area," said Pieralisi. "Here in northern Mississippi, we have a specific set of weed problems, but another region of the country might have a different set."
Lowe added, "Another limitation of our current images is that they show healthy weeds. In the field, a weed may be run over or blemished, so the camera would need to recognize weeds that are not in perfect form. Building the database with more varied images will help the camera recognize what it may actually analyze in the field."
An additional benefit to building a robust database is that it would help weed scientists track and manage species from year to year.
"If we could continue to build out the database and log the species we see in the field each year, maybe we could answer some questions about the effectiveness of or resistance to herbicides," Chesser said. "We always want to capture the data and use it to solve problems on the ground."
This research is funded by Cotton Incorporated and the Mississippi Agricultural and Forestry Experiment Station.
Farmers are looking to this technology to alleviate labor costs and optimize their production systems. In the future, our tool could potentially be integrated into an autonomous system that would traverse a field by itself, further reducing labor costs.
Dr. daniel chesser
Behind the Science
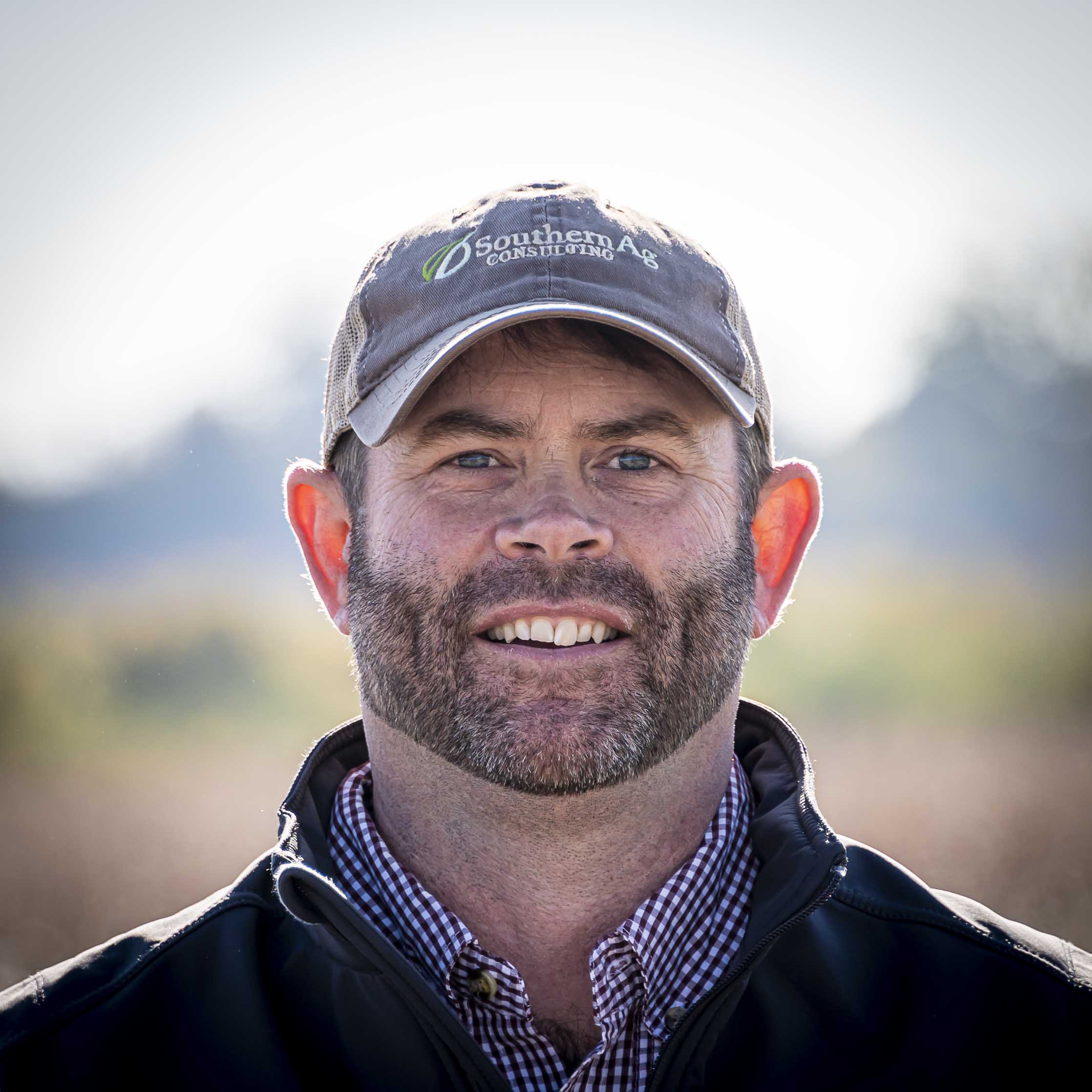
Daniel Chesser
Assistant Professor
Education: B.S., Agricultural Engineering, Technology, and Business; M.S., Ph.D., Agricultural Science, Mississippi State University
Years At MSU: 22
Focus: Applied research and development of precision agricultural machinery and agrotechnical systems
Passion At Work: My passion is improving sustainability and production efficiencies of cropping systems through the development of applied engineering and technology solutions that foster environmental stewardship and positively impact economic returns for farmers and industry alike.
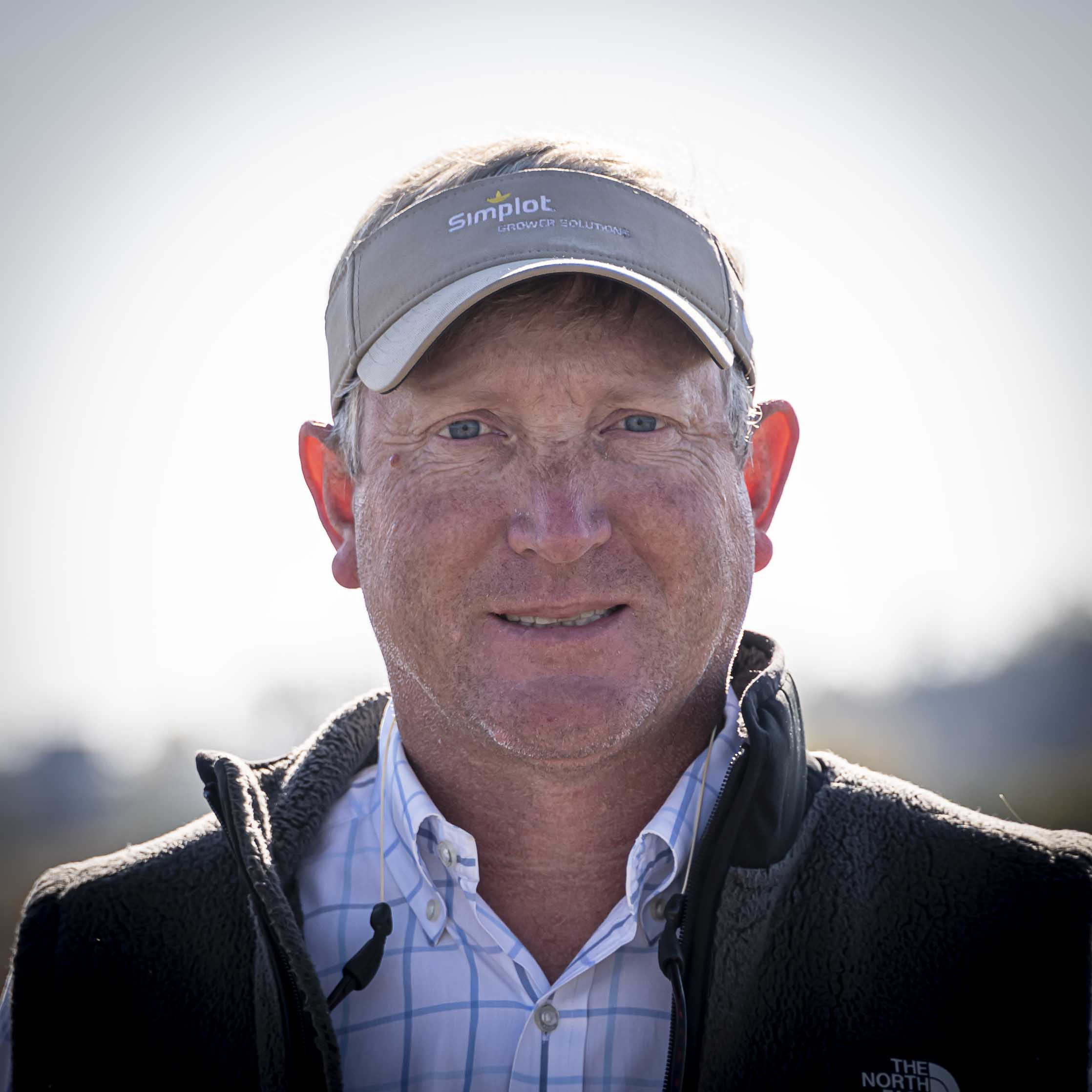
Wes Lowe
Assistant Professor
Education: B.S., M.S., Ph.D., Agricultural Engineering Technology & Business, Mississippi State University
Years At MSU: 17
Focus: Develop and apply automated and autonomous precision agriculture technology to streamline plant and animal production systems, providing farmers with tools to enhance efficiency, productivity, and profitability
Passion At Work: My research develops solutions that aid our farmers in maximizing their potential productivity, creating sustainable production systems
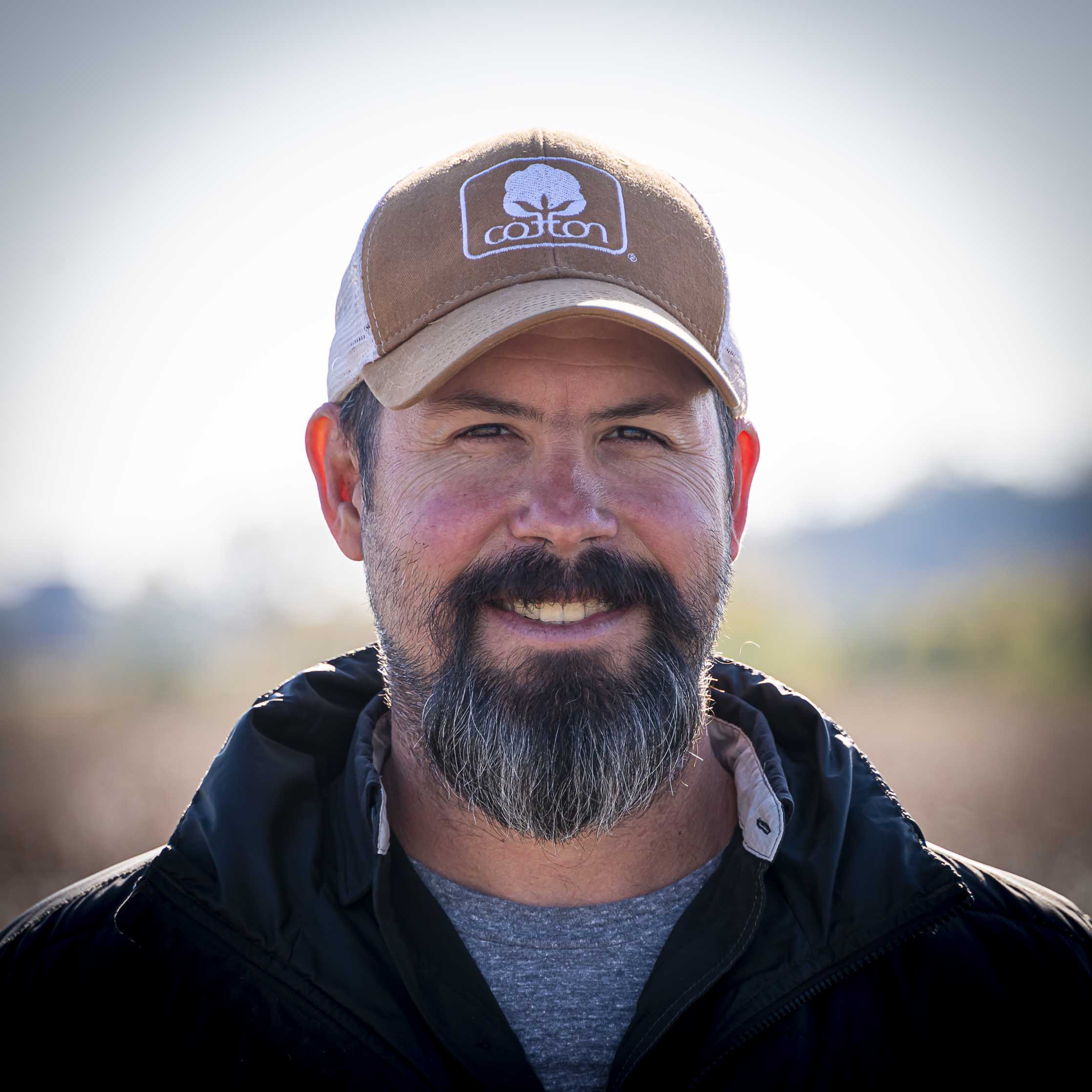
Brian Pieralisi
Assistant Professor, Extension Cotton Specialist
Education: B.S. Agricultural Pest Management, Mississippi State University; MBA, Delta State University; Ph.D., Agronomy, Mississippi State University
Years At MSU: 7
Focus: Cotton variety testing
Passion At Work: Finding solutions to problems facing growers by refining agronomic practices including irrigation/drainage, planting populations, row spacing, cover crops, and soil fertility.